A multistakeholder perspective on computable phenotypes for generating real-world evidence: can they really be standardized and reused?
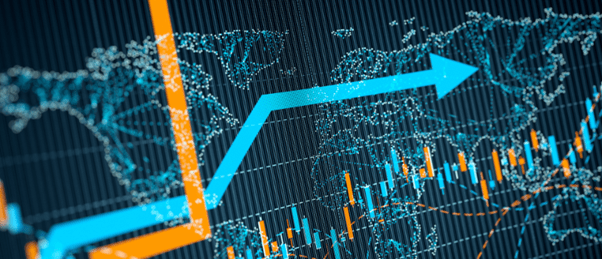
In this interview with Rachel Richesson (University of Michigan), Elise Berliner (Cerner Enviza), Kevin Haynes (Janssen) and David Carrell (Kaiser Permanente Washington Health Research Institute), we delve into the topic of their recent panel discussion at ISPOR 2022 (DC, US, 15–18 May 2022); ‘Standing up computable phenotypes for generating real-world evidence: what are computable phenotypes and can they really be standardized and reused?’. We discuss the process of standardization, key issues concerning computable phenotypes and how to conduct standardization to avoid perpetuating inequalities.
Biographies:
Rachel Richesson is an informaticist and professor of Learning Health Sciences at the University of Michigan Medical School (MI, USA).
Elise Berliner is the Global Senior Principal of Real-World Evidence Strategy at Cerner Enviza (MO, USA) and was formerly the Director of the Technology Assessment Program at the Center for Outcomes and Evidence in the Agency for Healthcare Research and Quality (AHRQ; MD, USA).
Kevin Haynes is the Associate Director of Epidemiology at Janssen (Beerse, Belgium).
David Carrell is an associate investigator at Kaiser Permanente Washington Health Research Institute (WA, USA) and has contributed substantially to phenotyping efforts in the eMERGE Network and the FDA Sentinel Initiative (MD, USA). His current work in Sentinel is focused on attempting to improve the accuracy of FDA phenotypes for medical product safety surveillance by incorporating structured and unstructured electronic health record data, including data derived by natural language processing, and the application of machine learning methods to better model the complex relationships in real-world data.
What are computable phenotypes and why did you assemble this panel?
Elise Berliner: Computable phenotypes are definitions of clinical conditions, outcomes and exposures that can be implemented in real-world data sources, such as electronic health record (EHR) data or medical claims data. Computable phenotypes are derived from clinical phenotypes – the clinical descriptions of the patient population and outcomes that patients experience.
In my work at AHRQ in technology assessment and systematic review, I had seen how the lack of harmonization of clinical phenotypes in research studies leads to the inability to compare results across studies and prevents clear conclusions that can be utilized for decision-making by patients, providers and policymakers.
Now that I am working with real-world data (RWD), I see how variation in computable phenotypes can add another layer of uncertainty to the validity and interpretation of studies derived from RWD. Therefore, I was happy to see that the FDA noted the benefits of standardized computable phenotypes for data sharing in the September 2021 guidance document on Assessing Electronic Health Records and Claims data for Real World Evidence. However, I had many questions about how standardization of computable phenotypes could or would work, so I invited the experts to explore these issues with me at an ISPOR panel.
Can phenotype definitions truly be standardized?
Rachel Richesson: There are great efficiencies and potential benefits for utilizing ‘standard’ condition-specific phenotype definitions. However, standardizing phenotype definitions is challenging. Different studies have different needs. Different organizations collect data and utilize coding systems in different ways. Who decides which definition is the ‘standard’ computable phenotype for, as an example, diabetes? What if your organization does not have access to certain types of data that are required for the phenotype? Or if a new study has a greater need for specificity than the standard definition performs?
Different study requirements and data availability will inevitably lead to variation and multiplicity of phenotype definitions for a given condition. One approach to standardization is to reduce the variation by encouraging and enabling the re-utilization of existing high quality and validated computable phenotypes.
To do this at scale will require platforms for people to post phenotype definitions with descriptive metadata to help potential users identify and assess existing definitions for their purposes. These metadata need to describe the purpose, development approach, specifications and performance of the definition. Capturing and reporting this metadata will require efforts on the part of phenotype developers and it is not clear at present if there is any incentive to do this. Further, building these platforms, such as PheKB, and developing metadata and reporting requirements will take resources. But the looming – and limiting – question is: who will support and regulate this?
Is it possible to re-utilize a phenotype from an outside organization? Do you still need to validate locally?
Kevin Haynes: There are challenges in creating reusable phenotypes that can operate on heterogeneous EHR data with different levels of data quality, population representation and data capture. I am generally in the camp that these algorithms are not that transportable across space, meaning the database, and time, both within the database types of EHRs and claims. Although I’m a little more persuaded that an Aetna-validated algorithm will work in an Anthem claims environment than, for example, an EHR phenotype algorithm in health system A on Cerner works in health system B on Epic.
However, there is concern that coding practices and EHR systems vary over time with changes in coding practices and data feed availability. Additionally, there is the challenge of matching a research question to the data that is available. Researchers must balance incident vs prevalent vs exacerbation of a prior event, all of which are further complicated by the variable follow-up time across individuals and variable data quality across data resources.
That said, do I move phenotypes around various databases? Yes. I always have my eye on methods to validate with medical records or through novel methods to further understand the study-specific data quality needs of RWD to address real-world evidence.
Is there another way to speed the development and validation of phenotypes that work in local populations?
David Carrell: The transportability of a given, published phenotype algorithm is limited, or at least more limited than many people would like to acknowledge. I also believe computable phenotypes need to be re-validated in each setting, cohort or time era, with the last two also applying within one setting. There are just too many ways that setting/cohort/era can make algorithms perform differently.
Because of this, I think one useful strategy for phenotype ‘reusability’ is to focus on the reusability and scalability of the algorithm development process itself, rather than the product of that process, the algorithm. Making the development process itself so efficient that it becomes feasible to replicate the entire development process in each setting is one of the appeals of the more automated approaches, like PheNorm and PheCAP.
Some epidemiologists and statisticians find the idea that each site in a multi-site study would develop and utilize its own version of an algorithm very distasteful, but I suspect that the lack of such concerns when all sites are utilizing the exact same algorithm is due to a fair amount of wishful thinking and illusion about the idiosyncrasies and heterogeneity of data across sites, cohorts and era.
The automated approaches have limitations of course—including requiring access to clinical notes—and will certainly not work for all phenotypes. However, the ‘reusable phenotype algorithm problem’ is so big and diverse that there won’t be a single solution for all phenotypes.
Disclaimers:
Rachel Richesson, Elise Berliner, Kevin Haynes and David Carrell have no disclaimers to declare.
The opinions expressed in this feature are those of the interviewee/author and do not necessarily reflect the views of The Evidence Base®.