Realizing ‘fit-for-purpose’ real-world data
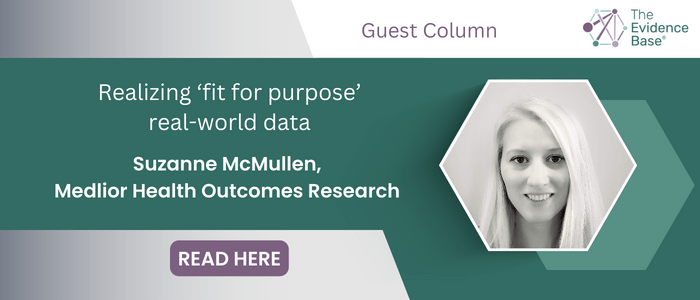
Routinely collected, real-world data (RWD) relating to epidemiology, patient and clinical characteristics, health outcomes, quality of life, treatment patterns, and resource utilization/costs are increasingly in demand to generate real-world evidence (RWE) to inform healthcare decision-making. The demand stems from a widespread urgency to better understand the burden of a disease and unmet needs of patient populations, to evaluate the real-world safety, effectiveness, and value of innovative (and often costly) health technologies. However, identifying RWD sources, and assessing whether the data captured is ‘fit-for-purpose’ from a regulatory/reimbursement perspective, is a key challenge that Medlior Health Outcomes Research Ltd (Medlior) has been addressing for over ten years.
The RWE problem
Researchers producing RWE are often confronted with identifying appropriate data sources based on a specific question or uncertainty that needs to be addressed. The utility of RWE to inform decisions, and ultimately improve patient care, relies largely on whether the data source is ‘fit-for-purpose’ and whether the provenance and quality of data is trusted by decision-makers [1–4]. Selecting the best available data source to address these areas of uncertainty is one of the most pivotal first steps in designing an impactful RWE study.
Researchers and manufacturers will often look for existing sources of RWD to gain substantial time- and cost-efficiencies compared to prospective data collection methods. A challenge in this approach is identifying a relevant data source among the numerous datasets that may be available worldwide, like finding the needle in a haystack. Administrative and claims-based datasets, for example, are robust datasets commonly leveraged by researchers and manufacturers to inform regulatory/reimbursement decisions; however, these datasets may lack important variables identifying specific populations (e.g., rare disease) or clinical outcomes (e.g., disease progression/severity).
In summary, identifying RWD that is ‘fit-for-purpose’ is more challenging than it may seem. Therefore, it is critical to the success of the study and acceptability of the evidence to first evaluate each dataset for suitability and consider if there are better, alternative data options available.
The RWE solution: Phase 1 – RWD identification
Because identifying appropriate data sources can be tricky and time-consuming, Medlior developed Real World Radar™, a subscription-based digital directory of international data sources. Real World Radar lists over 2200 unique datasets from 36 jurisdictions around the world and continues to expand with new datasets added each month.
You may also be interested in:
Real World Radar: the first online directory of real-world data
The vision for Real World Radar is to add value to the research community by fostering synergistic RWE studies while improving time, resource, and cost efficiencies. Real World Radar also promotes collaboration by providing a platform for researchers to identify other organizations/data sources in their therapeutic areas of interest. Offering a convenient solution, Real World Radar supports the identification of ‘fit-for-purpose’ RWD to improve the acceptance of RWE studies by decision-makers.
Real World Radar has keyword search functionality, to quickly identify relevant RWD sources. In addition, the integration with PubMed allows researchers to view citations associated with that database and understand how the data has been leveraged in prior studies. Click here to view an online demo.
In addition to Real World Radar, Medlior offers database mapping services, to help clients identify and extract publicly available information on relevant RWD sources in their therapeutic area of interest.
The RWE solution: Phase 2 – feasibility
Once potential data sources have been identified, the next step is assessing the feasibility of leveraging the data for research. Medlior’s feasibility assessments address the following objectives:
- ‘Fit-for-Purpose’ – Is this data source appropriate for specific research questions/objectives?
- Ethical and Legal Requirements – What are the ethical and legal requirements? For example, can this data be utilized for secondary research?
- Logistics – What is the process for requesting, accessing, analyzing, and reporting the data?
- Budget – What are the fees associated with utilizing this data source?
- Timelines – What are the timelines for working with this data source?
Additionally, several ‘fit-for-purpose’ objectives assess the elements in each data source relating to three key factors:
- Data Relevance
- Region/population covered
- Content and structure of the data
- Estimated sample size obtainable
- Length of follow-up
- Data Provenance
- Original purpose and methods of data collection
- Details of how the dataset was created (e.g., linkage, pooling)
- Data preparation (e.g., cleaning, mapping of the raw data)
- Data Quality
- Reliability of the data
- Extensiveness
- Coherence
At Medlior, feasibility assessments align with guidance documents from international decision-making bodies on how to select an appropriate, feasible data source specific to a study’s objectives:
- The Food and Drug Administration (FDA) [3] and Canada’s Drug and Health Technology Agency (CADTH) instruct researchers on assessing data fitness for decision-making, and CADTH’s Guidance for Reporting Real-World Evidence, released in 2023 [4], provides researchers with clear guidelines on reporting the fitness of data for use.
- The National Institute for Health and Care Excellence (NICE)’s Real-World Evidence Framework, published in 2022, includes a Data Suitability Assessment Tool (DataSAT) [2].
- The European Medicines Agency (EMA)’s Data Quality Framework for EU Medicines Regulation, published in 2023, provides clear details of how to assess data quality [1].
- Another resource for evaluating the fitness of a data source is the recently published ATRAcTR (Authentic Transparent Relevant Accurate Track-Record) [5], featured here in The Evidence Base.
The bottom line
The guidance from international regulatory and health technology assessment bodies is clear: ‘fit-for-purpose’ RWD is essential for RWE to be accepted by decision-makers. Leveraging Real World Radar to identify RWD sources, paired with a feasibility assessment to ensure relevance and logistical requirements are aligned, can increase the impact and success of your RWE study, efficiently and conveniently.
Author
Suzanne McMullen, Vice President of Research and Innovation, Medlior Health Outcomes Research
As Vice President of Research and Innovation at Medlior Health Outcomes Research Ltd, Suzanne oversees research activities and pursues innovative solutions to advancing the methodology and applications of HEOR. Suzanne has over 15 years of research experience in both commercial and public sector organizations, including roles with ICON Plc, Oxford Outcomes, Vancouver Coastal Health and the Centre for High-Throughput Biology.
For more information on RWR or to set-up a live demo, contact Medlior on [email protected].
Sponsorship for this Guest Column was provided by Medlior Health Outcomes Research.