Ask the Experts: advancing high-quality real-world data to support evolving uses of real-world evidence
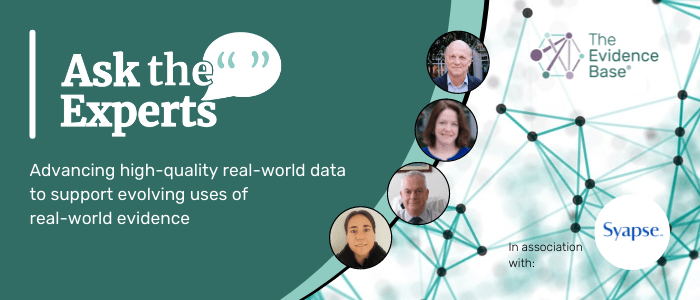
In this ‘Ask the Experts’ feature, in association with Syapse (CA, USA), we explore some of the potential benefits or novel insights real-world data may provide, as well as challenges associated with generating evidence from these data, and what methods may be used to develop and assess scalable and high-quality real-world datasets, by posing some key questions to a panel of experts with diverse experiences.
Our experts for this instalment are Dr Thomas Brown (Syapse), Dr Orazio Caffo (Santa Chiara Hospital, Trento, Italy), Manuela Di Fusco (Pfizer, NY, USA) and Dr Alissa Winzeler-Cotton (Syapse). Take a look at the full discussion below to hear their insights!
Questions
Jump to:
Broadly speaking, what benefits or novel insights can real-world data provide?
What is the importance of understanding the source of the real-world data you are using?
What methods may be used to develop and assess scalable and high-quality real-world datasets?
Could you discuss some of the key challenges associated with working with real-world data and generating evidence from these? How may evidence generation processes be validated?
What has the COVID-19 pandemic taught us about the potential of real-world data/real-world evidence?
How do you see the role of real-world evidence evolving over the next 5 years?
Meet the experts
Dr Thomas Brown
Dr Thomas Brown is the Chief Medical Officer at Syapse. Dr Brown is an internationally renowned oncologist and cancer researcher, and is an affiliate faculty member of the Institute for Systems Biology (WA, USA). Prior to arriving at Syapse, Dr Brown was Executive Director of the Swedish Cancer Institute (WA, USA). Dr Brown has served in leadership roles on the faculties of several academic cancer centers, including at the University of Arizona (AZ, USA), MD Anderson (TX, USA) and Duke University (NC, USA). He has also served as an Executive Officer within the Southwest Oncology Group (SWOG) (USA).
Dr Brown holds a Bachelor of Arts degree from Johns Hopkins University (MD, USA) and an MBA from Rice University (TX, USA). A graduate of the Medical College of Virginia (VA, USA), he completed an internal medicine residency at the University of Florida (FL, USA) and a fellowship in medical oncology at the Johns Hopkins University School of Medicine. His clinical, research and administrative efforts have focused on early phase clinical trials, precision medicine, healthcare policy and global medicine. Dr Brown has served on numerous institutional, national and international committees and boards. Among numerous honors over his career, Dr Brown was selected as a Jones Scholar while at Rice University and has been selected for inclusion in Best Doctors of America beginning in 1994.
Dr Orazio Caffo
Dr Orazio Caffo currently serves as Chief of the Medical Oncology Department at Santa Chiara Hospital (Trento, Italy). Dr Caffo is a member of several professional organizations, including the American Society of Clinical Oncology (VA, USA), the European Society for Medical Oncology (Lugano, Switzerland), the International Association for the Study of Lung Cancer (CO, USA), the Italian Medical Oncology Association (Milan, Italy) and the Italian Society of Urological Oncology (Bologna, Italy). He is also a member of the Executive Board of the Italian Society of Urological Oncology.
Dr Caffo’s research primarily focuses on genitourinary and lung cancers, with interests in medical treatments – either alone or in combination with radiation therapy – and quality of life assessments.
Dr Caffo chairs several Italian multicentric trials, is a Principal Investigator on several international trials and has authored or co-authored more than 170 articles that have been published in peer-reviewed journals.
Manuela Di Fusco
Manuela Di Fusco is a Director in the Health Economics and Outcomes Research, Patient and Health Impact team at Pfizer Inc. (NY, USA).
Di Fusco’s academic background is in economics and health technology assessment. Over the past 10 years, her research interests have been focused on health economics and outcomes research projects across multiple drugs and phases of drug development.
Dr Alissa Winzeler-Cotton
Dr Alissa Winzeler-Cotton is the Vice President of Strategy, Research and Medicine at Syapse. Dr Winzeler-Cotton is a versatile healthcare leader who leverages her unusual background to bridge the gap between science and business strategy. She holds an A.B. in Biochemical Sciences from Harvard University (MA, USA) and a PhD in Developmental Biology from Stanford University (CA, USA).
Prior to joining Syapse, Dr Winzeler-Cotton spent over 5 years as a management consultant in the healthcare practice at McKinsey & Company (CA, USA), where she worked with large health systems, life science companies and health insurance companies to overcome a wide variety of strategic, operational and organizational effectiveness challenges. At Syapse, she and her team have led major strategic initiatives that have helped to transform Syapse from a precision medicine workflow company into a real-world evidence company, including guiding strategic priorities for data augmentation and curation, building proprietary real-world data science algorithms, developing and managing strategic research collaborations, and bringing life science partners into the Syapse Learning Health Network.
1. Broadly speaking, what benefits or novel insights can real-world data provide?
Dr Thomas Brown: The potential benefits of using real-world data to generate meaningful insights are tremendous. Specifically, high-quality real-world data have the potential to address limitations of classical clinical trials, and to augment post-marketing analyses of the safety and efficacy of newly approved drugs. In this manner, real-world data can impact the US FDA’s regulatory efficiency and the ability to rapidly bring new drugs to patients. Real-world data can also support the healthcare ecosystem in addressing urgent healthcare challenges, such as the ongoing COVID-19 pandemic, in a timely manner.
Real-world data have the potential to support stakeholders across the healthcare continuum by:
- Helping clarify how therapies perform in real-world populations that are underrepresented in clinical trials – e.g., in underserved communities, patients with comorbid conditions and the aging population;
- Helping providers identify and close gaps in care to ensure that every patient receives the best chance of a good outcome.
Dr Orazio Caffo: Real-world data are able to expand data provided from randomized controlled trials, mainly to help answer questions that are not usually covered by marketing-driving studies. This is the case, for example, of data on sequential use of agents that had received their market license when the enrolled patients were quite different from those observed in daily clinical practice, due to the introduction of other drugs not available at the time of trial plan.
Manuela Di Fusco: The insights generated from real-world data can complement and supplement the knowledge gained from other study designs. Real-world data can add valuable information on disease epidemiology, unmet needs, treatment patterns, and the comparative effectiveness and safety of treatment options in large and diverse populations, under conditions not studied in clinical trials, over long observation durations and/or relative to multiple alternative interventions.
“The potential benefits of using real-world data to generate meaningful insights are tremendous,”
– Dr Thomas Brown.
Real-world data also allow for a broad set of outcomes to be assessed and can be used efficiently to address research questions that require long follow-up periods, large patient numbers, or that cannot be studied in clinical trials for ethical or feasibility reasons.
A variety of variables and outcomes not often studied in clinical trials can be assessed in real-world analyses such as adherence, dosing, patient-reported outcomes, resource utilization and costs, across relevant subgroups.
Real-world data have broad applications: they can be leveraged to calibrate economic evaluations, track the quality of care, inform clinical decision-making, support outcome-based coverage agreements and support future research, among others.
Dr Alissa Winzeler-Cotton: Real-world data and real-word evidence have the potential to help stakeholders improve outcomes for patients in a variety of ways, for example:
- Characterizing populations not well served by current therapeutic options, to prioritize unmet needs in drug development;
- Reducing costs, time and risks for clinical trials targeting molecular subpopulations;
- Providing supportive evidence to accelerate regulatory approvals for molecularly targeted therapies;
- Identifying gaps between the evidence curve and the adoption curve and supporting providers in closing those gaps;
- Developing evidence of safety and real-world effectiveness for patients not well represented in clinical trials.
2. What is the importance of understanding the source of the real-world data you are using?
Dr Thomas Brown: Not all oncology care settings are the same. The oncology provider landscape includes integrated community health systems, independent practices and academic centers. As one evaluates datasets to use in research, it is critical to understand the provider landscape.
At present, most oncology real-world data come from independent oncology practices and academic cancer centers. Providers in these settings have notable differences in incentives and practice patterns. In generating real-world evidence, one needs to understand the inherent biases within a given data set.
“…a detailed understanding of the quality, validity and reliability of the real-world data source is therefore critical, to inform the viability of a study,”
– Manuela Di Fusco.
An example of this is that real-world data from independent cancer specialty practices will likely not include comprehensive non-cancer and/or pre-cancer care data. An understanding of medical history prior to a cancer diagnosis, baseline comorbidities, inpatient and outpatient care, along with non-cancer related care, is essential to accurately assessing a patient’s care journey. Data from large integrated health systems are more likely to render this comprehensive picture.
Dr Orazio Caffo: This knowledge is needed in order to help increase our confidence in the reliability and robustness of the real-world data. Data from administrative sources are robust and sure, but they are frequently unable to answer to clinical questions. The most useful data are provided by clinical records, but, in my opinion, the capturing modalities should be clearly defined, mainly to avoid the use of incomplete information.
Manuela Di Fusco: Real-world data can be collected in multiple ways, from multiple stakeholders, for different purposes and populations; a detailed understanding of the quality, validity and reliability of the real-world data source is therefore critical, to inform the viability of a study.
A ‘feasibility assessment’ stage is an important early step for evaluating the availability and validity of information needed to conduct a real-world study leveraging a certain dataset. Some of the key aspects to look at during such a feasibility analysis include: sample size; the potential for missing data and miscoding; the representativeness of the population and care spectrum (e.g., inpatient, outpatient); and the definition and measurement of endpoints and subgroups.
Moreover, a review of the data capture and cleaning processes can inform the overall reliability of the data source for research purposes. These thorough assessments of a data source can support a decision on whether a certain dataset can be effectively used to answer a specific research question, what design and analytic approaches are feasible, and what novel insights could be generated.
Dr Alissa Winzeler-Cotton: When it comes to real-world data, understanding sourcing is critical. Because real-world data are developed from a specific set of providers and their patients, the practice patterns are specific to those providers. Integrated community health systems deliver approximately 51% of cancer care in the USA. Therapy adoption models extrapolating from trends seen in independent practices (approximately 32% of the market) are likely to miss the mark considerably, given the differences between these provider groups.
Sourcing of individual dataset components is also extremely important. All datasets have inherent biases and limitations. Leveraging a multisource approach to data collection can help fill in the gaps and support both a more complete and reliable dataset; however, one must maintain provenance of the data throughout. That is, one needs to keep track of which data are being brought in, how they are merged and what went into the final composite dataset.
“In my opinion, a checklist of minimum characteristics for a data set can help in defining the quality level of the real-world data derived from the set,”
– Dr Orazio Caffo.
As an example, we recently published a paper describing our mortality composite score [1], which brings together six different sources of mortality data into a final opinion on the mortality information for each patient. In the paper, we documented biases in completeness across sex, race, age and socioeconomic status that occurred in each of the individual sources. If one is using mortality data sourced primarily from digitized obituaries, for example, then it is important to realize that you will be more likely to miss dates of death for female, Black, Asian and younger individuals. These missing dates of death may impact the shape of the survival curves one develops from the data being used. Fortunately, our paper demonstrated that by bringing these six sources together into a composite score, we were able to overcome these biases. The paper highlights, however, that understanding the data sources you are using – and what their inherent biases are – is critical to developing trustworthy real-world evidence.
3. What methods may be used to develop and assess scalable and high-quality real-world datasets?
Dr Thomas Brown: Of course, in order to trust the answers obtained from real-world data, it is essential to ensure the data are accurate and complete. Too often, it is assumed that all real-world data are equivalent in quality. One way to assure quality is to obtain data from multiple sources, thus ensuring completeness. At the same time, properly arbitrating conflicting data for the same data element is important, to ensure accuracy.
Cancer care has become incredibly complex, as a myriad of new biomarkers, associated targeted therapies and immunotherapies have been introduced, with patient care becoming highly individualized. Leveraging real-world data and evidence can help bring clarity to this complexity, but only if the real-world data are of high quality.
Dr Orazio Caffo: In my opinion, a checklist of minimum characteristics for a data set can help in defining the quality level of the real-world data derived from the set. As there is a broad range of fields where real-world data may be applied, it is very difficult to identify more rigid criteria for developing and collecting real-world data that would be applicable across all fields.
Manuela Di Fusco: Real-world data are being increasingly used to fill in evidence needs, inform clinical decision-making, support health economics research and advance the understanding of drug development.
“…all datasets have inherent biases and limitations,”
– Dr Alissa Winzeler-Cotton.
However, they come from a variety of data sources, tied to diverse care delivery systems, heterogeneous data collection processes, and collected data can vary in breadth and depth. As a consequence, a multitude of study designs and analytic approaches raise questions upon the reliability of real-world data, their quality and to what extent real-world evidence can be interpreted consistently. Acceptability of real-world-data by regulators for decision-making is limited and mainly exploratory.
Some ad-hoc initiatives to support the development of high-quality and scalable datasets include the development and adoption of common protocols and data models (e.g., the Observational Medical Outcomes Partnership common data model), the adoption of technology-friendly user interfaces for simplified and systematic data capture, ad-hoc efforts on quality checking, cleaning and maintenance processes (some of which might explore the application of artificial intelligence), as well as best practice sharing and alignment across multiple institutions around a culture of high-quality data collection.
Dr Alissa Winzeler-Cotton: In a recent blog post [2], we emphasize how important it is to understand the steps that companies are taking to develop high-quality real-world data. As was discussed earlier, all datasets have inherent biases and limitations. In order to ensure that the data are both scalable and of high enough quality to provide trustworthy insights, companies developing real-world evidence typically use some combination of three different approaches.
The first is the integration and harmonization of multiple scaled sources. The second is the use of human intelligence to transform a messy, often unstructured, patient record into structured data. Finally, most companies in the industry tout the use of artificial intelligence in some form, to enhance either the data or the insights that are derived from them. All three approaches have both value and limitations, and, when evaluating a real-world data source, it is particularly important to understand what is being done under the hood to get the data into shape for high-quality real-world evidence to be developed, how the data are being measured, and what the bar is for quality.
4. Could you discuss some of the key challenges associated with working with real-world data and generating evidence from these? How may evidence generation processes be validated?
Dr Thomas Brown: As we consider ‘regulatory-grade evidence’ and the establishment of standards-of-care, it is important to approach research using real-world data with the same hypothesis-driven and scientifically rigorous approach as is utilized in a prospective clinical trial, essentially emulating a clinical trial using real-world data.
“Too often, it is assumed that all real-world data are equivalent in quality,”
– Dr Thomas Brown.
Prospective development of a clear hypothesis, establishment of eligibility criteria, definition of index dates to include the window of time for observations, definition of the study endpoints and stipulation of the biostatistical methodology are all critical to the successful leveraging of real-world data. Given the strong evidence-based approach to modern cancer care, with daily care closely informed by clinical trial methodology and results, the field of oncology is uniquely positioned for the leveraging of real-world data and evidence, to advance the understanding of disease and development of new therapies.
Dr Orazio Caffo: The different approaches to collection and analysis can generate real-world data and evidence that are rarely fully comparable and combinable. To help address this, the native real-world data should be made available for further elaborations and analyses, to overcome limitations due to differences in collection modalities etc.
Clearly, the available infrastructure at the level of a single center is not sufficient to guarantee data sharing of isolated sets. Big data analysis could overcome this limitation.
Manuela Di Fusco: Multiple challenges are associated with working with real-world data. These include limitations of the data source(s), and the design and methodological approaches used, which impact the overall applicability of findings.
Certain clinical parameters may not be captured (e.g., some laboratory values), generating potential for missing data. Just like with clinical trials, real-world analyses can be subject to a lack of statistical power from a design and analysis standpoint. Depending on the nature of the dataset and the data capture process, other challenges may relate to coding errors, the representativeness of the population and care spectrum, and the measurement and ascertainment of endpoints.
Real-world data analyses are not randomized, leading to potential for bias, as evidenced by treatment groups often showing differences in baseline characteristics. Despite adjusting for patient baseline demographic and clinical characteristics, confounding may still occur, due to unmeasured variables and lack of randomization.
“Once one has ensured that the real-world data being used are of high quality, it takes another layer of expertise to transform those data into meaningful evidence,”
– Dr Alissa Winzeler-Cotton.
Finally, since the factors that influence treatment choice in clinical practice may also influence clinical outcomes, and due to the complex nature of both known and unknown variables in the data, concrete cause-and-effect type conclusions cannot be made. So, while clinical studies are able to infer causality, real-world analyses can only highlight associations between treatments and outcomes.
Multiple resources exist to support researchers’ awareness of key challenges and guide research [3–7].
Dr Alissa Winzeler-Cotton: Once one has ensured that the real-world data being used are of high quality, it takes another layer of expertise to transform those data into meaningful evidence. Data may exist for a particular part of the patient journey, but it often requires additional algorithms to transform those data elements into useful information. For example, how do you take all of the various therapies that a patient receives and transform those into simply ‘lines of therapy’, which is how oncologists think about the patient treatment journey? Additionally, the outcome measures that are typically leveraged in a clinical trial setting can never be exactly replicated. Even something as seemingly straightforward as real-world overall survival requires making methodological choices that can affect the results.
These – and other – challenges have inspired the FDA to actively partner with Syapse and other real-world evidence thought leaders to further advance the real-world data science that is used to transform these data into information. The FDA is working directly with real-world evidence companies such as ours to both develop new methodologies and test the value of the insights the data provide, sometimes by comparing with comparable studies using clinical trial data.
Oncology is at the forefront of real-world evidence development for a number of reasons, but there is growing interest in real-world evidence in other areas as well. Many of the methodologies developed will be specific to the field of oncology (e.g., lines of therapy, time to next therapy), but others may transfer across fields more readily (e.g., a history of pneumonitis).
5. What has the COVID-19 pandemic taught us about the potential of real-world data/real-world evidence?
Dr Thomas Brown: In any unexpected public healthcare crisis, with millions of lives at risk, there is initially insufficient time to await the results of either prospective epidemiologic studies or classical randomized clinical trials of therapeutic interventions. Real-world data can offer the recency to support more rapid understanding of a previously unknown disease, such as COVID-19. The rapid and innovative development of COVID-19 vaccines by life science companies, in partnership with governmental and research entities, has been commendable. This novel disease, and a new vaccine so quickly distributed, is a proving ground for how to use real-world data during an urgent public health challenge. Whether this is to help further understanding of acute complications, effectiveness of therapies, vaccine outcomes, or long-term effects of COVID-19, a clinical trial only reveals a fraction of the impact of this pandemic.
“Real-world data have played a critical role in advancing the understanding of COVID-19 and answering critical questions with regard to this novel disease, at an unprecedented speed,”
– Manuela Di Fusco.
Real-world data – and resulting real-world evidence – can render a fuller picture of the unfolding pandemic, to include the impact on children or the significance of asymptomatic carriers, etc. When scaled and produced at high quality, with accuracy and completeness, real-world data can highlight evidence and insights otherwise inaccessible.
Dr Orazio Caffo: The pandemic has been the most important occasion in the health field to test the real usefulness and potency of real-world data. Without real-world data, it would be very difficult to address practical questions concerning infection outcomes and management. On the other hand, the different aims of the real-world data reports confirmed the difficulties in summarizing their evidence, which frequently led to different conclusions – for example, regarding the relationship between androgen-deprivation therapy for prostate cancer and COVID-19 outcomes.
Manuela Di Fusco: Real-world data have played a critical role in advancing the understanding of COVID-19 and answering critical questions with regard to this novel disease, at an unprecedented speed. Numerous real-world data research activities for COVID-19 encompass global, large-scale research projects that leverage collaborations and information sharing among diverse stakeholders and prompt the use of common data models to generate key insights. An example of these initiatives is the COVID-19 Evidence Accelerator project.
Dr Alissa Winzeler-Cotton: Real-world evidence proved itself to be incredibly valuable in the early days of the pandemic. In a fireside chat we recently hosted with Dr Amy Abernethy, former Principal Deputy Director of the FDA, Dr Abernethy highlighted the value of the insights garnered into the descriptive epidemiology of COVID-19 from real-world data.
The data provided a rapid window into who was getting sick and how sick they were getting – that is, what patient demographics and comorbidities impacted disease frequency, severity and outcomes. In a global pandemic, rapid answers to even these simple questions can be valuable in ensuring that the most at-risk patients are identified and treated appropriately.
6. How do you see the role of real-world evidence evolving over the next 5 years?
Dr Thomas Brown: A growing number of collaborations, involving a range of stakeholders including the FDA, are underway with the goal of standardizing the use of real-world evidence. My hope is that these collaborations further establish standards of quality to include further validation of outcome endpoints most appropriate for real-world data. I believe that real-world evidence can be routinized for regulatory filings, creating clearer paths for leveraging real-world evidence in New Drug Applications. I also hope that we will soon leave behind the notion that real-world evidence and classical prospective clinical trials are in perpetual competition, and begin to recognize the important nexus of the two.
“…the native real-world data should be made available for further elaborations and analyses, to overcome limitations due to differences in collection modalities…”
– Dr Orazio Caffo.
Due largely to the efforts of the FDA, new drugs are coming to market more rapidly and more efficiently. This places more importance on supplementary real-world evidence as a component of New Drug Applications, as well as more importance on leveraging real-world evidence to further elucidate post-marketing safety and efficacy characteristics of new drugs. Real-world evidence will also allow for observational research that follows cohorts of patients – some having been in clinical trials – into the future to understand long-term impacts, as in the case of COVID-19 in patients with cancer. This latter topic is of particular interest as we can also use real-world data to understand how delays in cancer screenings and tests ultimately impact outcomes and the severity of cancer cases.
Dr Orazio Caffo: As long as guidelines from scientific societies will consider real-world evidence by providing a real role for real-world data, I think it will become one of the main sources of evidence.
Manuela Di Fusco: I believe that real-world evidence will continue to play a significant role for public health. It will fill in evidence gaps and advance our understanding of diseases, patient profiles, outcomes and long-term use of treatments in clinical practice. The field will also continue to evolve and generate powerful and actionable insights informing healthcare decision-making.
Dr Alissa Winzeler-Cotton: First, as the field evolves, standards will become more well-established for how to develop high-quality real-world evidence and stakeholders, including the FDA, will become more comfortable with evaluating real-world evidence against those standards. Getting to this level of comfort in evaluating real-world evidence is one of the reasons the FDA has established research collaboration agreements with real-world evidence companies, such as ours.
Second, as our CEO, Ken Tarkoff, recently discussed [8], real-world evidence has great potential not only because it can add to the clinical literature, but also because it can be brought directly back into the clinic to be acted on.
As providers see increasing value in using real-world data to drive the highest quality clinical care for their patients, the incentive to enhance the documentation of clinical care to further support this value increases. This creates a virtuous cycle in which the providers who invest in more consistent documentation (e.g., of Eastern Cooperative Oncology Group performance scores) derive greater and greater value from real-world data in treating their patients.
Disclaimers:
The opinions expressed in this feature are those of the interviewees/authors and do not necessarily reflect the views of The Evidence Base® or Future Science Group.
This feature was produced in association with Syapse.
Manuela Di Fusco is a paid employee of Pfizer Inc., with ownership of stock in Pfizer Inc. The views expressed are her own.
Orazio Caffo has received speaker/advisor grants from Astellas, AstraZeneca, Bayer, Janssen, MSD, Pfizer and Sanofi.
[1] Lerman MH, Holmes B, St Hilaire D et al. Validation of a mortality composite score in the real-world setting: overcoming source-specific disparities and biases. JCO Clin Cancer Inform. 5, 401–413 (2021).
[2] Ken Tarkoff. Can you trust your real-world data? https://syapse.com/company/blog/can-you-trust-your-real-world-data (Accessed 19 May 2021).
[3] Motheral B, Brooks J, Clark MA et al. A checklist for retrospective database studies – report of the ISPOR Task Force on Retrospective Databases. Value Health 6(2), 90–97 (2003).
[4] Berger ML, Mamdani M, Atkins D, Johnson ML. Good research practices for comparative effectiveness research: defining, reporting and interpreting nonrandomized studies of treatment effects using secondary data sources: the ISPOR Good Research Practices for Retrospective Database Analysis Task Force Report – Part I. Value Health 12(8), 1044–1052 (2009).
[5] Berger ML, Sox H, Willke RJ et al. Good practices for real-world data studies of treatment and/or comparative effectiveness: recommendations from the joint ISPOR-ISPE Special Task Force on real-world evidence in health care decision making. Pharmacoepidemiol Drug Saf 26(9), 1033–1039 (2017).
[6] Dreyer NA, Bryant A, Velentgas P. The GRACE checklist: a validated assessment tool for high quality observational studies of comparative effectiveness. J Manag Care Spec Pharm 22(10), 1107–1113 (2016).
[7] Public Policy Committee, International Society of Pharmacoepidemiology. Guidelines for good pharmacoepidemiology practice (GPP). Pharmacoepidemiol Drug Saf 25(1), 2–10 (2016).
[8] Ken Tarkoff. Bringing real world evidence into the care setting. https://www.managedhealthcareexecutive.com/view/bringing-real-world-evidence-into-the-care-setting (Accessed 19 May 2021).
Syapse (CA, USA) is a real-world evidence company dedicated to extinguishing the fear and burden of serious disease by advancing real-world care. By integrating complete, longitudinal and continuously updated real-world data, we can provide unique insights into patients’ care journeys. Our advantage derives from a decade of partnerships within the Syapse Learning Health Network of innovation-driven community health systems, life sciences, regulators and molecular labs. Syapse empowers healthcare organizations to work together in active, transparent partnerships, constantly assembling the most relevant real-world data, generating purposeful real-world evidence and transforming insights into a better patient experience you can actually see. Together, we are working toward a future in which all cancer patients have access to the best precision care.